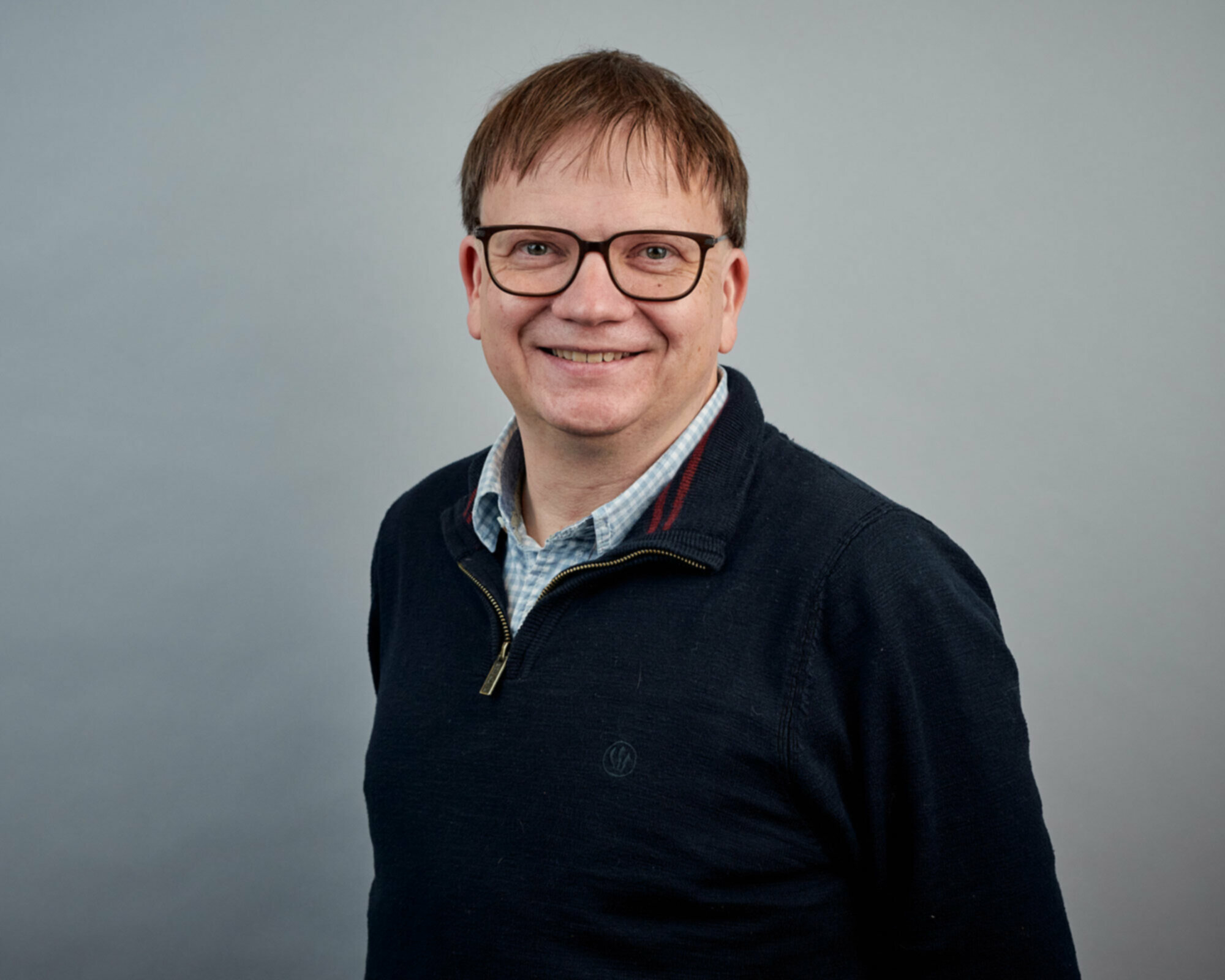
Rufus Grig
Chief Technology & Strategy Officer, Kerv Group|Kerv
Have a question?
Get in touchPublished 09/01/25 under:
At its core, AI is powered by data. Machine learning algorithms rely on vast amounts of data to learn, adapt and make accurate predictions that can revolutionise day-to-day business functions.
So, while AI can achieve incredible results, if the data is incorrect, the results will be too.
The most recent episode of Learning Kerv dived into the real-world applications of Generative AI, focusing on why data is so important!
Our AI expert host, Rufus Grig, CTO of Kerv Group, was joined by Kyle Ansari, CTO of Kerv Collaboration & Compliance, David Groves Product Director at Kerv, and Dawn Kelso, Data Solution Director at Kerv, to share their experiences and insights into how AI is transforming various aspects of business operations.
Let’s look at some of the key takeaways from their discussion!
Operational AI in Compliance
Generative AI in Compliance is a game-changer. AI can categorise and analyse alarms to rule out ‘false positive’ alarms so that genuine issues are being dealt with as quickly and as efficiently as possible.
Kyle Ansari estimated that Kerv was dealing with three to four thousand false positive alarms a day from businesses, without any way to determine what was genuine other than combing through them one by one. Kyle’s solution was to create an AI model, integrated with Compliance Cloud, that can do that hard work in seconds, by using generative AI to manage compliance cloud services and catch the real issues that need to be addressed.
Therefore, using AI can impact a dramatic change in operation. Kyle mentioned, ‘We reduce our intervention of tickets by 97%’, which has improved the accuracy of issue detection, even, at times, identifying problems before Microsoft did.
AI in Customer Service
AI has proven essential for customer service, particularly in multilingual environments. David Groves, the developer of CX Translate, highlights the importance of AI automated translation in contact centre systems, as he states this rids the need for large multilingual staff or for agents to spend time translating themselves.
However, this only works if the translation is accurate, David stresses that the AI model requires data to manage idioms and context from languages so the customer is fully understood, and their issue can be resolved quickly!
Putting automated translation at the heart of contact centres, initially on web chat and WhatsApp, and eventually email, can streamline communication to enable the agent to solve a customer’s issue quicker and more efficiently.
David comments on varying accuracy of translations depending on the language, noting that Western European languages tend to perform. However, this is an evolving technology and AI model, more integration of context and glossaries has, and will continue to, improved the quality of translations.
The overall result of using AI in contact centres has radically changed customer service, making customer interactions smoother and more effective, not only freeing up agent’s time but leading to more customer satisfaction.
Data Quality and AI
Data quality is the key to successful use of AI in Business Applications. Dawn Kelso says, ‘Your outcomes are only ever going to be as good as the data,’ highlighting the issue that poor data quality can lead to biased or inaccurate AI predictions.
Accurate, relevant and up-to-date data, as well as correct labeling and classification, are all important parts of ensuring your data quality is as high as possible.
Data is the backbone of successful use cases of AI, and it comes in two primary forms: labelled and unlabelled. Labelled data includes input-output pairs, where the output is known and used to train the model in supervised learning.
Unlabeled data, on the other hand, lacks this explicit output, making it suitable for unsupervised learning where the model identifies patterns and structures within the data.
Both types of data are critical for training robust AI models as they allow the AI model to learn and adapt to ultimately provide a more specific and unique outcome.
Dawn also comments on the need to have a human involved in this part of AI implementation, especially when managing sensitive information.
Tony Leary, a guest on a previous episode of Learning Kerv, highlighted the importance of data security by commenting,
Security is about keeping data safe, while privacy relates to our ability to exercise choices on how data is used and processed
To ensure data security, this is one area that needs to be monitored and reviewed by humans to ensure what AI is outputting is accurate, relevant and secure.
Future of Generative AI
Looking ahead, the potential of generative AI only continues to grow. Kyle hints at upcoming developments in compliance that could further reduce manual effort and improve efficiency. “There’s a whole stack of development we have planned for next year,” he teases, although we’ll have to wait for the exciting new applications!
David and Dawn also see vast opportunities for AI to enhance various business functions, from customer service to data management. As AI models evolve and improve, their applications will become even more integral to business operations.
Want to learn more about AI in Business Applications? Listen to the latest episode of Learning Kerv to explore all the practical applications that Gen AI can provide! As businesses continue to explore and implement AI solutions, the lessons shared by our experts will be crucial in guiding successful AI integration. Listen now!
Have a question?
"*" indicates required fields